Unlocking the Power of Image Data Annotation
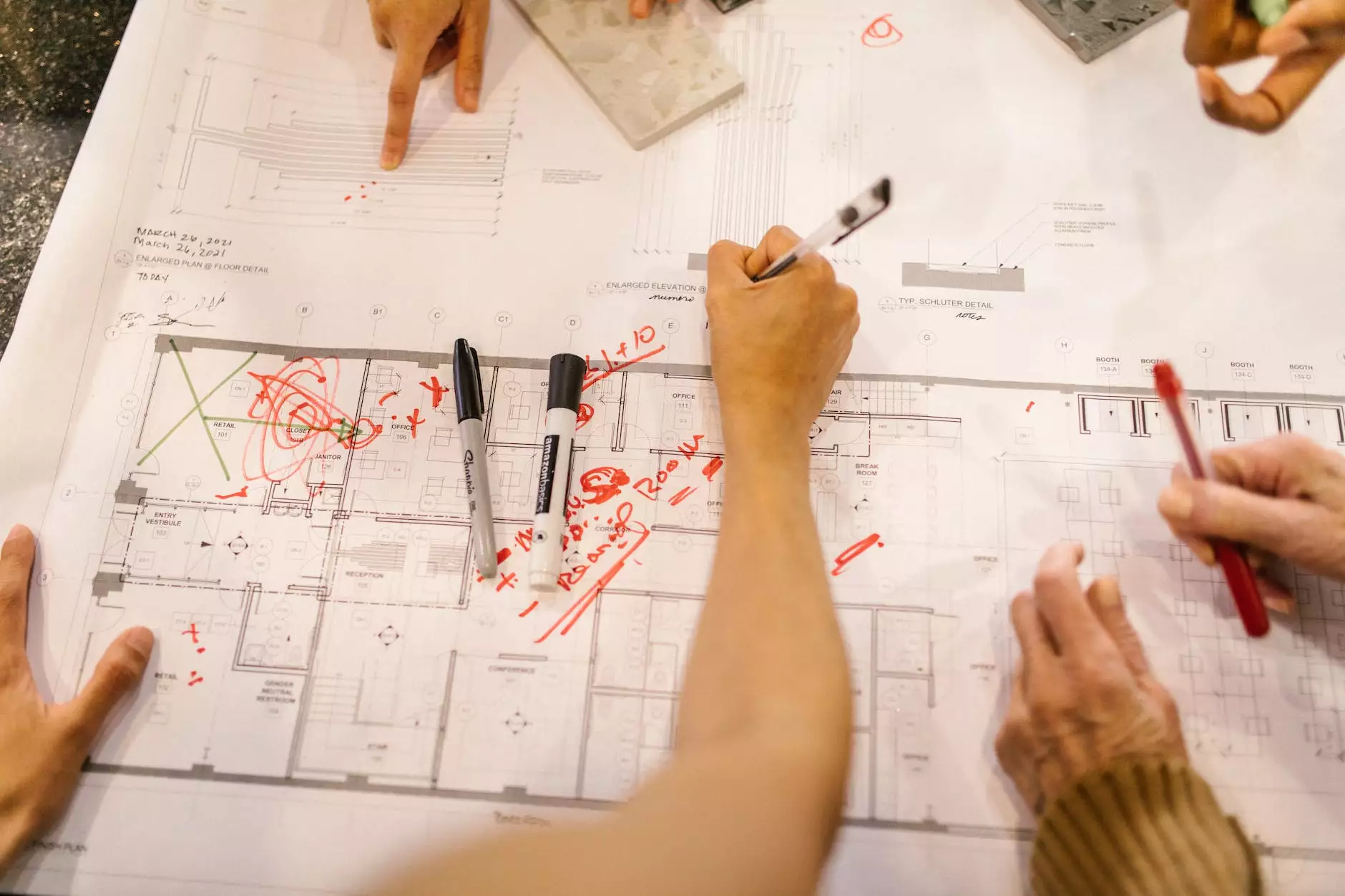
Image data annotation is fundamentally changing the landscape of artificial intelligence (AI) and machine learning (ML). As organizations seek to leverage data-driven decisions, the importance of high-quality annotated data cannot be overstated. Whether you're in the business of creating sophisticated computer vision applications or enhancing your existing processes, understanding the nuances of image data annotation will provide your organization with a competitive edge.
Understanding Image Data Annotation
At its core, image data annotation involves labeling images to create training data for AI models. This data helps machine learning algorithms learn to recognize patterns, classify objects, and make predictions based on visual content. The process can be manual or automated, but the goal remains the same: to produce high-quality data that drives accurate results.
Types of Image Data Annotation
There are various types of image data annotation techniques, each suited for different applications:
- Object Detection: Involves identifying and locating multiple objects within an image by drawing bounding boxes around them.
- Image Segmentation: This technique classifies each pixel in the image, providing a pixel-wise mask that distinguishes different objects.
- Image Classification: Labels an entire image with a single category or class, assisting in identifying what the image represents.
- Keypoint Annotation: Uses specific points on objects for tasks such as facial recognition or pose detection.
- Bounding Box Annotation: Involves encasing an object in a rectangle, providing essential information for object identification.
Why Is Image Data Annotation Important?
The significance of image data annotation spans across various industries, including:
1. Enhancing Machine Learning Accuracy
High-quality, accurately annotated images lead to improved performance of machine learning models. When models are trained on well-labeled datasets, they're more likely to generalize better and deliver precise predictions.
2. Driving Innovation in Computer Vision
As companies integrate AI into their products, image data annotation serves as the backbone for developments in computer vision. This innovation not only enhances user experience but also opens doors to new applications, from autonomous vehicles to facial recognition security systems.
3. Meeting Industry Requirements
Specific sectors such as healthcare, retail, and automotive often have stringent data regulations. Properly annotated data is crucial for compliance and ensures that applications meet quality standards.
Key Features of a Superior Data Annotation Tool
When selecting a data annotation platform, certain features can significantly enhance the annotation experience:
- User-Friendly Interface: An intuitive design allows team members to annotate images efficiently.
- Scalability: The tool should be able to handle increasing volumes of data without compromising on speed or quality.
- Collaboration Features: Tools that facilitate teamwork help ensure consistency and quality across the board.
- Quality Assurance Mechanisms: Implementing checks ensures that the final annotated data meets the required standards.
- Integration Capabilities: The ability to integrate with other software solutions can streamline workflows and improve productivity.
The Role of Keylabs.ai in Image Data Annotation
As a leader in the data annotation space, Keylabs.ai provides an innovative data annotation platform designed to meet the diverse needs of businesses:
1. Advanced Annotation Tools
Keylabs.ai offers a suite of advanced tools that simplify the image data annotation process. From automated annotation options powered by AI to manual tools that allow precise customization, our platform caters to all types of annotation tasks.
2. Quality and Consistency
Our quality assurance protocols guarantee that your data is annotated to the highest standards. We provide tools and best practices that optimize the annotation process, ensuring reliable outputs every time.
3. Expert Support
With a dedicated team of experts, Keylabs.ai ensures that you have the support you need at every stage of the annotation process. Whether it's training your team or providing technical assistance, we’re here to help.
Strategies for Effective Image Data Annotation
Implementing effective strategies for image data annotation can lead to higher quality outputs and improved efficiency:
1. Define Clear Guidelines
Establish comprehensive guidelines on how to annotate images to maintain consistency across different annotators. This documentation serves as a reference to ensure everyone is on the same page.
2. Utilize Pre-Annotated Data
Using pre-annotated datasets as benchmarks can help standardize the annotation process and improve training efficiencies for your team.
3. Invest in Training
Educate your team on best practices for data annotation. Regular training sessions can keep everyone updated on the latest trends and technologies.
4. Monitor and Evaluate
Continuously monitor the annotation outputs and provide feedback to improve quality. Setting up a review process builds a feedback loop that enhances overall data integrity.
Image Data Annotation Use Cases Across Industries
The applications of image data annotation are vast and varied. Here are some use cases across different sectors:
1. Healthcare
In healthcare, image data annotation is vital for training models that interpret medical images, such as MRI scans and X-rays. Annotated images assist in diagnosing diseases, enhancing radiological assessments, and personalizing treatment plans.
2. Automotive
Autonomous driving systems rely heavily on image data annotation. Labeled images are crucial for training vehicles to recognize pedestrians, traffic signals, and other essential elements on the road.
3. Retail
In the retail sector, image data annotation can enhance customer experiences through improved product recognition and recommendation systems. Annotated data allows for advanced features like visual search capabilities in apps.
4. Agriculture
Farmers and agri-tech companies use image data annotation for monitoring crop health and diagnosing issues through drone imagery. This can lead to better yields and more efficient resource use.
Future Trends in Image Data Annotation
As technology evolves, so too does the field of image data annotation. Here are upcoming trends to watch:
1. Automation and AI Integration
With the rise of AI, automated annotation tools are becoming more sophisticated. As these tools mature, they will significantly reduce the time and cost associated with data annotation.
2. Increased Demand for Augmented Reality (AR) Data
As AR technologies become mainstream, there's a growing demand for annotated datasets to train AR systems. Businesses must adapt to this trend by investing in tools and platforms to cater to this market.
3. Focus on Ethical AI
As data annotation plays a critical role in training AI models, the ethical implications of the data used will come under increasing scrutiny. Companies must ensure their annotation processes are ethically sound and create fair AI systems.
Conclusion: The Path Forward with Image Data Annotation
In today's data-driven world, mastering image data annotation is essential for businesses aiming to leverage AI and machine learning. With the right tools and strategies, companies can streamline their data annotation processes and position themselves for future success.
At Keylabs.ai, we understand that the quality of your data can make or break your AI projects. Our comprehensive data annotation tools and platforms are designed to empower your organization to harness the full potential of image data annotation. Partner with us to pave the way towards remarkable innovations in AI and machine learning.